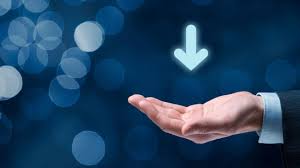

Finally, performance is evaluated through three different video benchmarks: own recorded videos in Madrid and Tehran (with different weather conditions at urban and interurban areas) and two well-known public datasets (KITTI and DETRAC). Then, Gaussian Mixture Models (GMM) are used to segment moving objects and to tackle car shadow effects, we apply a chromacity-based strategy. After removing perspective using Modified Inverse Perspective Mapping (MIPM), Hough transform is applied to extract road lines and lanes.
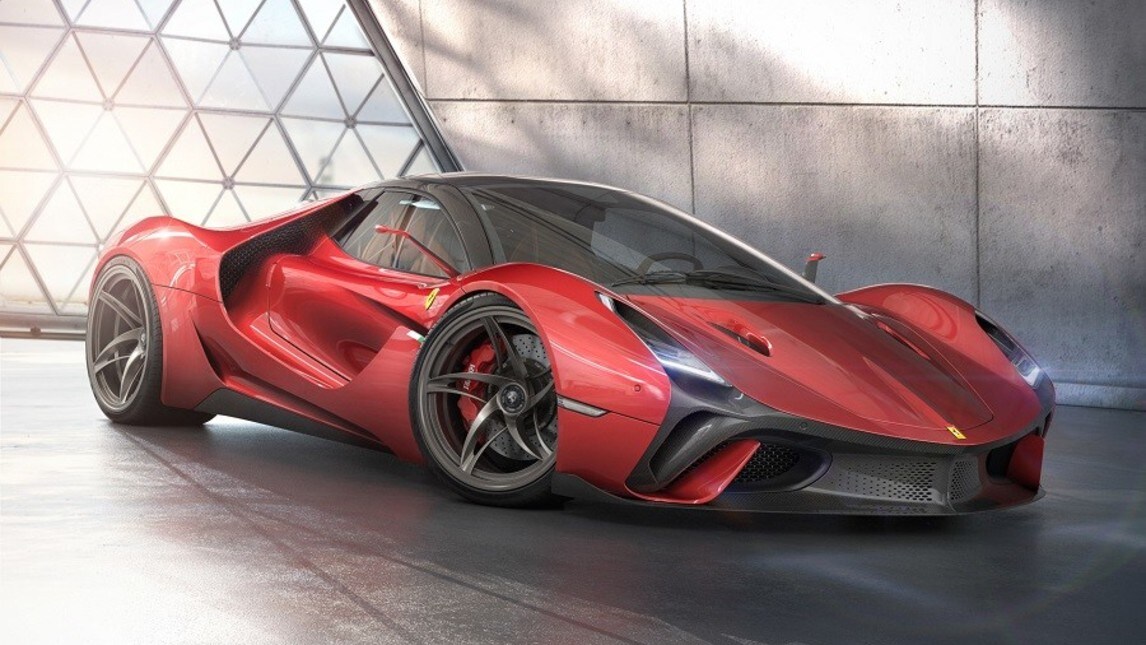
In this paper, we propose a new method to accurately segment and track vehicles.
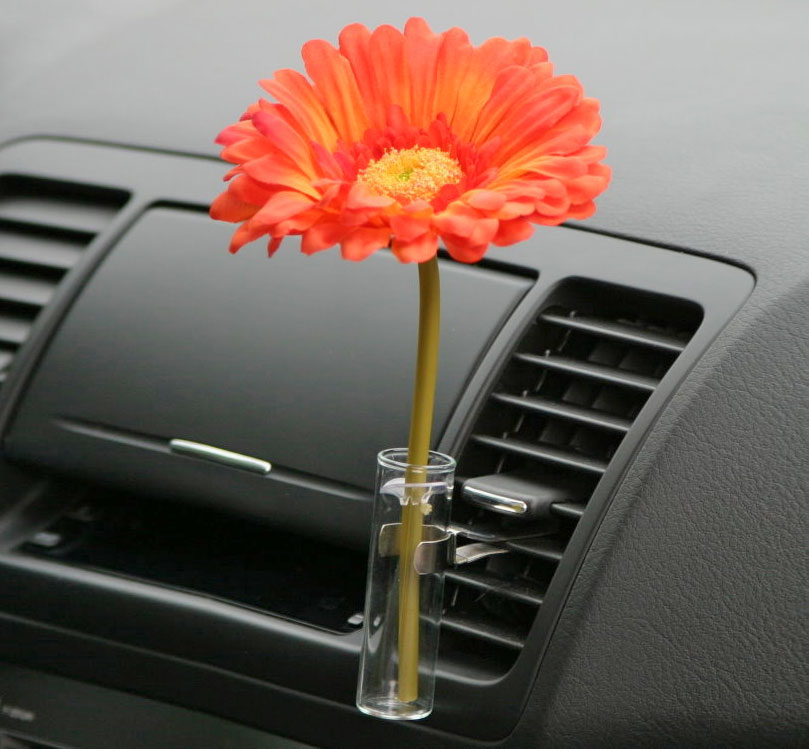
Conventional image-based traffic detection methods have difficulties acquiring good images due to perspective and background noise, poor lighting and weather conditions. Intelligent Transportation Systems (ITS) allow us to have high quality traffic information to reduce the risk of potentially critical situations. The obtained results show that the system proposed in this paper provides a counting rate higher than that of some existing methods. Our method can perform vehicle detection and classification by eliminating the influence of many factors on system efficiency. Our contribution consists of developing a feature-based counting system for vehicle detection and recognition under the conditions which present a challenge in recent systems, such as occlusions, and illumination conditions. The proposed system can measure the quantity and characteristics of traffic in real time based on three modules, segmentation, classification and vehicle counting. This paper presents a real-time management and control system which serve to analyze road traffic using a stationary camera. Road traffic video surveillance can help to resolve many problems which can influence road safety. It presents an important way for analysis of road traffic in highways. Today, Road traffic video surveillance becomes the centre of several concerns. At the point when one can detect and classify vehicles, then it is probable to more improve the flow of the traffic and even give enormous information that can be valuable for many applications in the future. Finally, some limitations shared between the systems are demonstrated, such as the definition of ROI, focusing on only one aspect of detection, and the variation of accuracy with quality of videos. Then a variety of algorithms and techniques developed to detect vehicles are discussed beside illustrating their advantages and limitations. First, we illustrate the concept of vehicle detection and discuss background subtraction for acquiring only moving objects. Generally, three steps are utilized to acquire traffic condition information, including background subtraction (BS), vehicle detection and vehicle counting. In this review, different automated VD systems have been surveyed, with a focus on systems where the rectilinear stationary camera is positioned above intersections in the road rather than being mounted on the vehicle. VD in road traffics has numerous applications in the transportation engineering field. The real-time processing is very critical to keep the appropriate functionality of automated or continuously working systems. Vehicle detection in videos combines image processing in real-time with computerized pattern recognition in flexible stages. One of the key advantages of traffic video detection is that it provides traffic supervisors with the means to decrease congestion and improve highway planning.

Traffic monitoring is an important element in the intelligent transportation system, which involves the detection, classification, tracking, and counting of vehicles. The need for intelligent facilities expanded because the total number of vehicles is increasing rapidly in urban zones. Vehicle detection (VD) plays a very essential role in Intelligent Transportation Systems (ITS) that have been intensively studied within the past years.
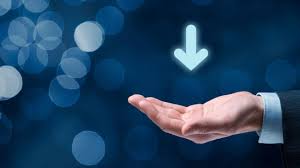